Spotting climate misinformation with AI requires expertly trained models
When classifying climate misinformation, general-purpose large language models lag behind models trained on expert-curated climate data.

Meta's Llama and Google’s Gemini lope within the again of proprietary ones within the job
Organizations that deserve to counter climate misinformation with AI deserve to herald specialists to data coaching of the items, a new ogle suggests.
rob dobi/Second/Getty Pictures Plus
Conversational AI chatbots are making climate misinformation sound extra credible, making it more durable to issue apart falsehoods from exact science. In response, climate specialists are using one of the main most same tools to detect counterfeit files on-line.
However through classifying unsuitable or misleading climate claims, extraordinary-cause huge language items, or LLMs — neutral like Meta’s Llama and OpenAI’s GPT-4 — lope within the again of items namely knowledgeable on knowledgeable-curated climate files, scientists reported in March on the AAAI Conference on Synthetic Intelligence in Philadelphia. Climate groups wishing to make utilize of recurrently on hand LLMs in chatbots and negate moderation tools to test climate misinformation deserve to fastidiously steal into consideration the items they utilize and herald relevant specialists to data the coaching direction of, the findings demonstrate.
When when put next with other varieties of claims, climate alternate misinformation is mostly “cloaked in unsuitable or misleading scientific files,” which makes it extra advanced for oldsters and machines to location the intricacies of climate science, says Erik Nisbet, a communications knowledgeable at Northwestern College in Evanston, In discouraged health.
To steal into consideration the items, Nisbet and his colleagues frail a dataset referred to as CARDS, which contains roughly 28,900 paragraphs in English from fifty three climate-skeptic websites and blogs. The paragraphs fall into five classes: “international warming is not any longer occurring,” “human greenhouse gases are no longer causing international warming,” “climate impacts are no longer bad,” “climate alternate suggestions obtained’t work” and “climate motion/science is unreliable.”
The researchers constructed a climate-particular LLM by retraining, or swish-tuning, OpenAI’s GPT-3.5-turbo3 on about 26,000 paragraphs from the same dataset. Then, the crew when put next the performance of the swish-tuned, proprietary model in opposition to 16 extraordinary cause LLMs and an openly on hand, small-scale language model (RoBERTa) knowledgeable on the CARDS dataset. These items categorized the final 2,900 paragraphs of misleading claims.
Nisbet’s crew assessed the items by scoring how wisely every categorized the claims into the staunch classes. The swish-tuned GPT model scored 0.84 out of 1.00 on the measure scale. The extraordinary cause GPT-4o and GPT-4 items had lower scores of 0.75 and 0.74, neutral just like the 0.77 ranking of the small RoBERTa model. This showed that together with knowledgeable feedback for the length of coaching improves classification performance. However the alternative nonproprietary items examined, neutral like these by Meta and Mistral, accomplished poorly, logging scores of up to best 0.28.
Here's an obvious , says Hannah Metzler, a misinformation knowledgeable from Complexity Science Hub in Vienna. The researchers faced computational constraints when using the nonproprietary items and couldn’t utilize extra highly efficient ones. “This shows that even as you happen to don’t get huge sources, which climate organizations obtained’t get, of direction there shall be concerns even as you happen to don’t deserve to make utilize of the proprietary items,” she says. “It shows there’s a enormous need for governments to create originate-source items and give us sources to make utilize of this.”
The researchers moreover examined the swish-tuned model and the CARDS-knowledgeable model on classifying unsuitable claims in 914 paragraphs about climate alternate printed on Facebook and X by low-credibility websites. The swish-tuned GPT model’s classifications showed excessive agreement with classes marked by two climate verbal substitute specialists and outperformed the RoBERTa model. However, the GPT model struggled to categorize claims in regards to the impact of climate alternate on animals and flowers, doubtlessly attributable to an absence of sufficient examples within the coaching files.
Yet every other ache is that generic items obtained't retain up with shifts within the certainty being shared. “Climate misinformation always varies and adapts,” Metzler says, “and it’s always gonna be advanced to lumber after that.”
Extra Tales from Science Knowledge on Synthetic Intelligence
What's Your Reaction?
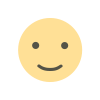
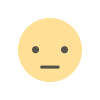
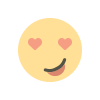
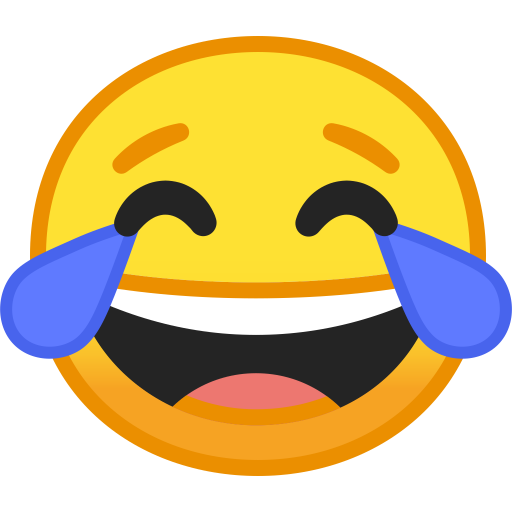
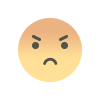
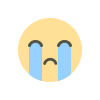
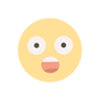